The Chicago-based American Medical Association is the country’s largest association of doctors and medical students. Now, for the first time ever, the organization has an African American woman as its president.
Dr. Patrice Harris, a psychiatrist from Atlanta, was inaugurated in June to the yearlong position. And while the AMA doesn’t represent all U.S. doctors, the organization is an influential advocacy group for a wide range of issues across the health and medical industries.
Looking ahead to her priorities over the next year, Harris says she hopes “to elevate the importance of mental health into overall health care, to elevate the importance of health equity, and making sure we have a diverse physician workforce … we need to work toward the faces of physicians matching the faces of our patients.”
As a lobbying group, the AMA has had an active presence in the health care debate, staunchly supporting the Affordable Care Act since it was passed under President Barack Obama in 2010.
The group has also held a longstanding opposition to single payer health care. But at its June meeting, the AMA House of Delegates only narrowly voted down a motion to overturn that policy.
“Certainly in our huge House of Delegates, you have a wide range of opinions, all towards getting to coverage for everyone,” Harris said. “But at the end of the day, after the debate, there’s a vote, and the vote this year has been to maintain our current policy.”
The AMA also recently waded into the abortion debate, filing a lawsuit earlier this month to block two laws in North Dakota the association says threaten the underlying trust between doctors and their patients.
The laws, Harris said, “compel physicians to provide information that is false, and misleading, and not science-based. And so that would be a violation of our duty to our patients … it is our obligation to give patients accurate information, and that is why we filed that lawsuit.”
Another issue Harris is prioritizing is health equity. That comes as a recent study in Chicago found a 30-year life expectancy gap between residents of the affluent Streeterville neighborhood and the low-income Englewood neighborhood on the South Side.
Harris says discrepancies like this aren’t just about access to doctors and hospitals.
“It is about your physical environment, whether you have access to healthy, nutritious foods. Even looking at some of the more structural policies in place, such as past discrimination and racism, all of those impact a person’s health, and that’s why you see those differences in zip code,” she said.
This comes at a time when diversity is a major challenge as well as a goal in all businesses.
First Black Female AMA President Talks Policy, Health Equity | Chicago News | WTTW: Meet Dr. Patrice Harris, the new leader of the Chicago-based American Medical Association, the country’s largest association of doctors and medical students.
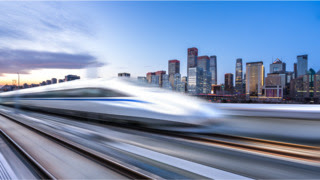
HEALTH TRAIN EXPRESS Mission: To promulgate health education across the internet: Follow or subscribe to Health Train Express as well as Digital Health Space for all the updates for health policy, reform, public health issues. Health Train Express is published several times a week.Subscribe and receive an email alert each time it is published. Health Train Express has been published since 2006.
Friday, July 19, 2019
Monday, July 8, 2019
This spray-on nanofiber 'skin' may revolutionize wound care
Imagine if bandaging looked a little more like, well, a water gun?
Shaped like a gun, Nanomedic’s SpinCare device emits a web of electrospun polymer nanofabric that stays put for weeks—no dressing changes required.
Israeli startup Nicast, has invented a new mechanical contraption to treat burns, wounds, and surgical injuries by mimicking human tissue. Shaped like a children’s toy, the lightweight SpinCare emits a proprietary nanofiber “second skin” that completely covers the area that needs to heal.
All one needs to do is aim, squeeze the two triggers, and fire off an electrospun polymer material that attaches to the skin.
The Nanomedic spray method avoids any need to come into direct contact with the wound. In that sense, it completely sidesteps painful routine bandage dressings. The transient skin then fully develops into a secure physical barrier with tough adherence. Once new skin is regenerated, usually between two to three weeks (depending on the individual’s heal time), the layer naturally peels off.
“You don’t replace it,” explains Nanomedic CEO Dr. Chen Barak. “You put it only once—on the day of application—and it remains there until it feels the new layer of skin healed.”
The SpinCare holds single-use ampoules containing Nanomedic’s polymer formulation. Once the capsule is firmly in place, one activates the device roughly eight inches towards the wound. Pressing the trigger activates the electron-spinning process, which sprays a web-like a layer of nanofibers directly on the wound.
The solution adjusts to the morphology of the wound, thereby creating a transient skin layer that imitates the skin structure’s human tissue. It’s a transparent, protective film that then allows the patient and doctor to monitor progress. Once the wound has healed and developed a new layer of skin, the SpinCare “bandage” falls off on its own.
The Nanomedic spray method avoids any need to come into direct contact with the wound. In that sense, it completely sidesteps painful routine bandage dressings. The transient skin then fully develops into a secure physical barrier with tough adherence. Once new skin is regenerated, usually between two to three weeks (depending on the individual’s heal time), the layer naturally peels off.
“You don’t replace it,” explains Nanomedic CEO Dr. Chen Barak. “You put it only once—on the day of application—and it remains there until it feels the new layer of skin healed.”
The product is already being tested in hospitals. In the coming year, following FDA clearance, Nanomedic plans to expand to emergency rooms, ambulances, military use, and disaster relief response like fire truck companies.
This spray-on nanofiber 'skin' may revolutionize wound care
Shaped like a gun, Nanomedic’s SpinCare device emits a web of electrospun polymer nanofabric that stays put for weeks—no dressing changes required.
Israeli startup Nicast, has invented a new mechanical contraption to treat burns, wounds, and surgical injuries by mimicking human tissue. Shaped like a children’s toy, the lightweight SpinCare emits a proprietary nanofiber “second skin” that completely covers the area that needs to heal.
All one needs to do is aim, squeeze the two triggers, and fire off an electrospun polymer material that attaches to the skin.
The Nanomedic spray method avoids any need to come into direct contact with the wound. In that sense, it completely sidesteps painful routine bandage dressings. The transient skin then fully develops into a secure physical barrier with tough adherence. Once new skin is regenerated, usually between two to three weeks (depending on the individual’s heal time), the layer naturally peels off.
“You don’t replace it,” explains Nanomedic CEO Dr. Chen Barak. “You put it only once—on the day of application—and it remains there until it feels the new layer of skin healed.”
The SpinCare holds single-use ampoules containing Nanomedic’s polymer formulation. Once the capsule is firmly in place, one activates the device roughly eight inches towards the wound. Pressing the trigger activates the electron-spinning process, which sprays a web-like a layer of nanofibers directly on the wound.
The solution adjusts to the morphology of the wound, thereby creating a transient skin layer that imitates the skin structure’s human tissue. It’s a transparent, protective film that then allows the patient and doctor to monitor progress. Once the wound has healed and developed a new layer of skin, the SpinCare “bandage” falls off on its own.
The Nanomedic spray method avoids any need to come into direct contact with the wound. In that sense, it completely sidesteps painful routine bandage dressings. The transient skin then fully develops into a secure physical barrier with tough adherence. Once new skin is regenerated, usually between two to three weeks (depending on the individual’s heal time), the layer naturally peels off.
“You don’t replace it,” explains Nanomedic CEO Dr. Chen Barak. “You put it only once—on the day of application—and it remains there until it feels the new layer of skin healed.”
The product is already being tested in hospitals. In the coming year, following FDA clearance, Nanomedic plans to expand to emergency rooms, ambulances, military use, and disaster relief response like fire truck companies.
This spray-on nanofiber 'skin' may revolutionize wound care
Saturday, July 6, 2019
Evaluation of a Remote Diagnosis Imaging Model vs Dilated Eye Examination in Detecting Macular Degeneration | Diabetic Retinopathy | JAMA Ophthalmology | JAMA Network
ADVANCES IN MEDICINE
JUST WHAT THE DOCTOR ORDERED:
IMPROVING PATIENT CARE WITH AI
Artificial Intelligence is transforming the world of medicine. AI can help doctors make faster, more accurate diagnoses. It can predict the risk of a disease in time to prevent it. It can help researchers understand how genetic variations lead to disease.
Although AI has been around for decades, new advances have ignited a boom in deep learning. The AI technique powers self-driving cars, super-human image recognition, and life-changing—even life-saving—advances in medicine.
Deep learning helps researchers analyze medical data to treat diseases. It enhances doctors’ ability to analyze medical images. It’s advancing the future of personalized medicine. It even helps the blind “see.”
“Deep learning is revolutionizing a wide range of scientific fields,” said Jensen Huang, NVIDIA CEO and co-founder. “There could be no more important application of this new capability than improving patient care.”
Three trends drive the deep learning revolution: more powerful GPUs, sophisticated neural network algorithms modeled on the human brain, and access to the explosion of data from the internet (see “Accelerating AI with GPUs: A New Computing Model”)
Community Medicine is a term used to describe medical conditions in a large population setting. It often involves the screening of large groups to select those with disease and provide appropriate treatment to avoid further complications. This involves an examination of large groups of patients. Often more than 100 persons will be examined with a positive finding of less than five in one hundred examinations. This is a massive undertaking when screening perhaps as much as 1000 or more persons. It is often not cost effective.
However, the development of image analysis, high-speed computing power, and deep learning machines can be trained to accomplish this task. Algorithms can be developed to digitize images (x-rays, CT scans, and photographs.
Artificial intelligence or machine learning is bringing a new powerful tool for rapid interpretation of medical images, such as chest x-rays, retinal fundus photography, and scans. Images of the skin can be analyzed for suspicious moles to rule out malignant melanoma rapidly. As the science matures there are sure to be significant cost savings as well as time.
Machine learning is dependent upon large data stores, and accuracy improves as images are added and curated by human beings (physicians). It is doubtful if AI will ever stand alone without human oversight.
A study of retinal fundus evaluation (as reported JAMA) using machine learning showed
Remote diagnosis imaging and a standard examination by a retinal specialist appeared equivalent in identifying referable macular degeneration in patients with high disease prevalence; these results may assist in delivering timely treatment and seem to warrant future research into additional metrics.
The study has shown equivalency in diagnosing age-related macular degeneration using ocular coherence tomography.
The use of deep learning has also been applied in dermatology to screen for malignant melanoma or other skin malignancy.
As radiology is inherently a data-driven specialty, it is especially conducive to utilizing data processing techniques. One such technique, deep learning (DL), has become a remarkably powerful tool for image processing in recent years. In this work, the Association of University Radiologists Radiology Research Alliance Task Force on Deep Learning provides an overview of DL for the radiologist. This article aims to present an overview of DL in a manner that is understandable to radiologists; to examine past, present, and future applications; as well as to evaluate how radiologists may benefit from this remarkable new tool. We describe several areas within radiology in which DL techniques are having the most significant impact: lesion or disease detection, classification, quantification, and segmentation.
Some are concerned that AI, or deep learning may replace human radiologists, however, this is unlikely to occur. But deep learning won’t be replacing radiologists anytime soon, Bratt explained, and one key reason for this is that deep neural networks (DNNs) are naturally limited by “the size and shape of the inputs they can accept.” But deep learning won’t be replacing radiologists anytime soon, Bratt explained, and one key reason for this is that deep neural networks (DNNs) are naturally limited by “the size and shape of the inputs they can accept.” A DNN can help with straightforward tasks reliant on a few images—bone age assessments, for instance, but they become less useful as the goal grows more and more complex. This limitation, Bratt explained, is related to the concept of long-term dependencies. Another issue related to DNNs is how easily they can fall apart when introduced to small changes. A DNN can be working perfectly after being trained on one institution’s dataset, for instance, but its performance suffers when it is introduced to new data from a new institution.
“This again reflects the fact that ostensibly trivial, even imperceptible, changes in input can cause catastrophic failure of DNNs, which limits the viability of these models in real-world mission-critical settings such as clinical medicine,” Bratt wrote.
In addition to evaluating images, DNN can be applied to other tasks.
MINING MEDICAL DATA FOR BETTER, QUICKER TREATMENT
Medical records such as doctors' reports, test results and medical images are a gold mine of health information. Using GPU-accelerated deep learning to process and study a patient's condition over time and to compare one patient against a larger population could help doctors provide better treatments.
BETTER, FASTER DIAGNOSES
Medical images such as MRIs, CT scans, and X-rays are among the most important tools doctors use in diagnosing conditions ranging from spine injuries to heart disease to cancer. However, analyzing medical images can often be a difficult and time-consuming process.
Researchers and startups are using GPU-accelerated deep learning to automate analysis and increase the accuracy of diagnosticians:
Imperial College London researchers hope to provide automated, image-based assessments of traumatic brain injuries at speeds other systems can't match.
Behold.ai is a New York startup working to reduce the number of incorrect diagnoses by making it easier for healthcare practitioners to identify diseases from ordinary radiology image data.
Arterys, a San Francisco-based startup, provides technology to visualize and quantify heart flow in the body using an MRI machine. The goal is to help speed diagnosis.
San Francisco startup Enlitic analyzes medical images to identify tumors, nearly invisible fractures, and other medical conditions.
GENOMICS FOR PERSONALIZED MEDICINE
Genomics data is accumulating in unprecedented quantities, giving scientists the ability to study how genetic factors such as mutations lead to disease. Deep learning could one day lead to what’s known as personalized or “precision” medicine, with treatments tailored to a patient’s genomic makeup.
Although much of the research is still in its early stages, two promising projects are:
A University of Toronto team is advancing computational cancer research by developing a GPU-powered “genetic interpretation engine” that would more quickly identify cancer-causing mutations for individual patients.
Deep Genomics, a Toronto startup, is applying GPU-based deep learning to understand how genetic variations lead to disease, transforming personalized medicine and therapies.
DEEP LEARNING TO AID BLIND PEOPLE
Nearly 300 million people worldwide struggle to manage such tasks as crossing the road, reading a product label, or identifying a face because they’re blind or visually impaired. Deep learning is beginning to change that.
Horus Technology, the winner of NVIDIA’s first social innovation award at the 2016 Emerging Companies Summit, is developing a wearable device that uses deep learning, computer vision, and GPUs to understand the world and describe it to users.
One of the early testers wept after trying the headset-like device, recalled Saverio Murgia, Horus CEO, and co-founder. “When you see people get emotional about your product, you realize it’s going to change people’s lives.”
Further DNN utilizes optical diffractive circuits in lieu of electrons
The setup uses 3D-printed translucent sheets, each with thousands of raised pixels, which deflect light through each panel in order to perform set tasks. By the way, these tasks are performed without the use of any power, except for the input light beam.
The UCLA team's all-optical deep neural network – which looks like the guts of a solid gold car battery – literally operates at the speed of light and will find applications in image analysis, feature detection, and object classification. Researchers on the team also envisage possibilities for D2NN architectures performing specialized tasks in cameras. Perhaps your next DSLR might identify your subjects on the fly and post the tagged image to your Facebook timeline. For now, though, this is a proof of concept, but it shines a light on some unique opportunities for the machine learning industry.
Dewinner of NVIDIA’s first social innovation award at the 2016 Emerging Companies Summit, is developing a wearable device that uses deep learning, computer vision, and GPUs to understand the world and describe it to users.
One of the early testers wept after trying the headset-like device, recalled Saverio Murgia, Horus CEO and co-founder. “When you see people get emotional about your product, you realize it’s going to change people’s lives.”
Evaluation of a Remote Diagnosis Imaging Model vs Dilated Eye Examination in Referable Macular Degeneration | Diabetic Retinopathy | JAMA Ophthalmology | JAMA Network: This study evaluates a retinal diagnostic device and compares its utility and outcomes with those of traditional eye examinations by retinal specialists for patients with potential retinal damage from diabetic retinopathy and age-related macular degeneration.
JUST WHAT THE DOCTOR ORDERED:
IMPROVING PATIENT CARE WITH AI
Artificial Intelligence is transforming the world of medicine. AI can help doctors make faster, more accurate diagnoses. It can predict the risk of a disease in time to prevent it. It can help researchers understand how genetic variations lead to disease.
Although AI has been around for decades, new advances have ignited a boom in deep learning. The AI technique powers self-driving cars, super-human image recognition, and life-changing—even life-saving—advances in medicine.
Deep learning helps researchers analyze medical data to treat diseases. It enhances doctors’ ability to analyze medical images. It’s advancing the future of personalized medicine. It even helps the blind “see.”
“Deep learning is revolutionizing a wide range of scientific fields,” said Jensen Huang, NVIDIA CEO and co-founder. “There could be no more important application of this new capability than improving patient care.”
Three trends drive the deep learning revolution: more powerful GPUs, sophisticated neural network algorithms modeled on the human brain, and access to the explosion of data from the internet (see “Accelerating AI with GPUs: A New Computing Model”)
Community Medicine is a term used to describe medical conditions in a large population setting. It often involves the screening of large groups to select those with disease and provide appropriate treatment to avoid further complications. This involves an examination of large groups of patients. Often more than 100 persons will be examined with a positive finding of less than five in one hundred examinations. This is a massive undertaking when screening perhaps as much as 1000 or more persons. It is often not cost effective.
However, the development of image analysis, high-speed computing power, and deep learning machines can be trained to accomplish this task. Algorithms can be developed to digitize images (x-rays, CT scans, and photographs.
Artificial intelligence or machine learning is bringing a new powerful tool for rapid interpretation of medical images, such as chest x-rays, retinal fundus photography, and scans. Images of the skin can be analyzed for suspicious moles to rule out malignant melanoma rapidly. As the science matures there are sure to be significant cost savings as well as time.
Machine learning is dependent upon large data stores, and accuracy improves as images are added and curated by human beings (physicians). It is doubtful if AI will ever stand alone without human oversight.
A study of retinal fundus evaluation (as reported JAMA) using machine learning showed
Remote diagnosis imaging and a standard examination by a retinal specialist appeared equivalent in identifying referable macular degeneration in patients with high disease prevalence; these results may assist in delivering timely treatment and seem to warrant future research into additional metrics.
The study has shown equivalency in diagnosing age-related macular degeneration using ocular coherence tomography.
The use of deep learning has also been applied in dermatology to screen for malignant melanoma or other skin malignancy.
As radiology is inherently a data-driven specialty, it is especially conducive to utilizing data processing techniques. One such technique, deep learning (DL), has become a remarkably powerful tool for image processing in recent years. In this work, the Association of University Radiologists Radiology Research Alliance Task Force on Deep Learning provides an overview of DL for the radiologist. This article aims to present an overview of DL in a manner that is understandable to radiologists; to examine past, present, and future applications; as well as to evaluate how radiologists may benefit from this remarkable new tool. We describe several areas within radiology in which DL techniques are having the most significant impact: lesion or disease detection, classification, quantification, and segmentation.
Some are concerned that AI, or deep learning may replace human radiologists, however, this is unlikely to occur. But deep learning won’t be replacing radiologists anytime soon, Bratt explained, and one key reason for this is that deep neural networks (DNNs) are naturally limited by “the size and shape of the inputs they can accept.” But deep learning won’t be replacing radiologists anytime soon, Bratt explained, and one key reason for this is that deep neural networks (DNNs) are naturally limited by “the size and shape of the inputs they can accept.” A DNN can help with straightforward tasks reliant on a few images—bone age assessments, for instance, but they become less useful as the goal grows more and more complex. This limitation, Bratt explained, is related to the concept of long-term dependencies. Another issue related to DNNs is how easily they can fall apart when introduced to small changes. A DNN can be working perfectly after being trained on one institution’s dataset, for instance, but its performance suffers when it is introduced to new data from a new institution.
“This again reflects the fact that ostensibly trivial, even imperceptible, changes in input can cause catastrophic failure of DNNs, which limits the viability of these models in real-world mission-critical settings such as clinical medicine,” Bratt wrote.
In addition to evaluating images, DNN can be applied to other tasks.
MINING MEDICAL DATA FOR BETTER, QUICKER TREATMENT
Medical records such as doctors' reports, test results and medical images are a gold mine of health information. Using GPU-accelerated deep learning to process and study a patient's condition over time and to compare one patient against a larger population could help doctors provide better treatments.
BETTER, FASTER DIAGNOSES
Medical images such as MRIs, CT scans, and X-rays are among the most important tools doctors use in diagnosing conditions ranging from spine injuries to heart disease to cancer. However, analyzing medical images can often be a difficult and time-consuming process.
Researchers and startups are using GPU-accelerated deep learning to automate analysis and increase the accuracy of diagnosticians:
Imperial College London researchers hope to provide automated, image-based assessments of traumatic brain injuries at speeds other systems can't match.
Behold.ai is a New York startup working to reduce the number of incorrect diagnoses by making it easier for healthcare practitioners to identify diseases from ordinary radiology image data.
Arterys, a San Francisco-based startup, provides technology to visualize and quantify heart flow in the body using an MRI machine. The goal is to help speed diagnosis.
San Francisco startup Enlitic analyzes medical images to identify tumors, nearly invisible fractures, and other medical conditions.
GENOMICS FOR PERSONALIZED MEDICINE
Genomics data is accumulating in unprecedented quantities, giving scientists the ability to study how genetic factors such as mutations lead to disease. Deep learning could one day lead to what’s known as personalized or “precision” medicine, with treatments tailored to a patient’s genomic makeup.
Although much of the research is still in its early stages, two promising projects are:
A University of Toronto team is advancing computational cancer research by developing a GPU-powered “genetic interpretation engine” that would more quickly identify cancer-causing mutations for individual patients.
Deep Genomics, a Toronto startup, is applying GPU-based deep learning to understand how genetic variations lead to disease, transforming personalized medicine and therapies.
DEEP LEARNING TO AID BLIND PEOPLE
Nearly 300 million people worldwide struggle to manage such tasks as crossing the road, reading a product label, or identifying a face because they’re blind or visually impaired. Deep learning is beginning to change that.
Horus Technology, the winner of NVIDIA’s first social innovation award at the 2016 Emerging Companies Summit, is developing a wearable device that uses deep learning, computer vision, and GPUs to understand the world and describe it to users.
One of the early testers wept after trying the headset-like device, recalled Saverio Murgia, Horus CEO, and co-founder. “When you see people get emotional about your product, you realize it’s going to change people’s lives.”
Further DNN utilizes optical diffractive circuits in lieu of electrons
The setup uses 3D-printed translucent sheets, each with thousands of raised pixels, which deflect light through each panel in order to perform set tasks. By the way, these tasks are performed without the use of any power, except for the input light beam.
The UCLA team's all-optical deep neural network – which looks like the guts of a solid gold car battery – literally operates at the speed of light and will find applications in image analysis, feature detection, and object classification. Researchers on the team also envisage possibilities for D2NN architectures performing specialized tasks in cameras. Perhaps your next DSLR might identify your subjects on the fly and post the tagged image to your Facebook timeline. For now, though, this is a proof of concept, but it shines a light on some unique opportunities for the machine learning industry.
Dewinner of NVIDIA’s first social innovation award at the 2016 Emerging Companies Summit, is developing a wearable device that uses deep learning, computer vision, and GPUs to understand the world and describe it to users.
One of the early testers wept after trying the headset-like device, recalled Saverio Murgia, Horus CEO and co-founder. “When you see people get emotional about your product, you realize it’s going to change people’s lives.”
Evaluation of a Remote Diagnosis Imaging Model vs Dilated Eye Examination in Referable Macular Degeneration | Diabetic Retinopathy | JAMA Ophthalmology | JAMA Network: This study evaluates a retinal diagnostic device and compares its utility and outcomes with those of traditional eye examinations by retinal specialists for patients with potential retinal damage from diabetic retinopathy and age-related macular degeneration.
Subscribe to:
Posts (Atom)